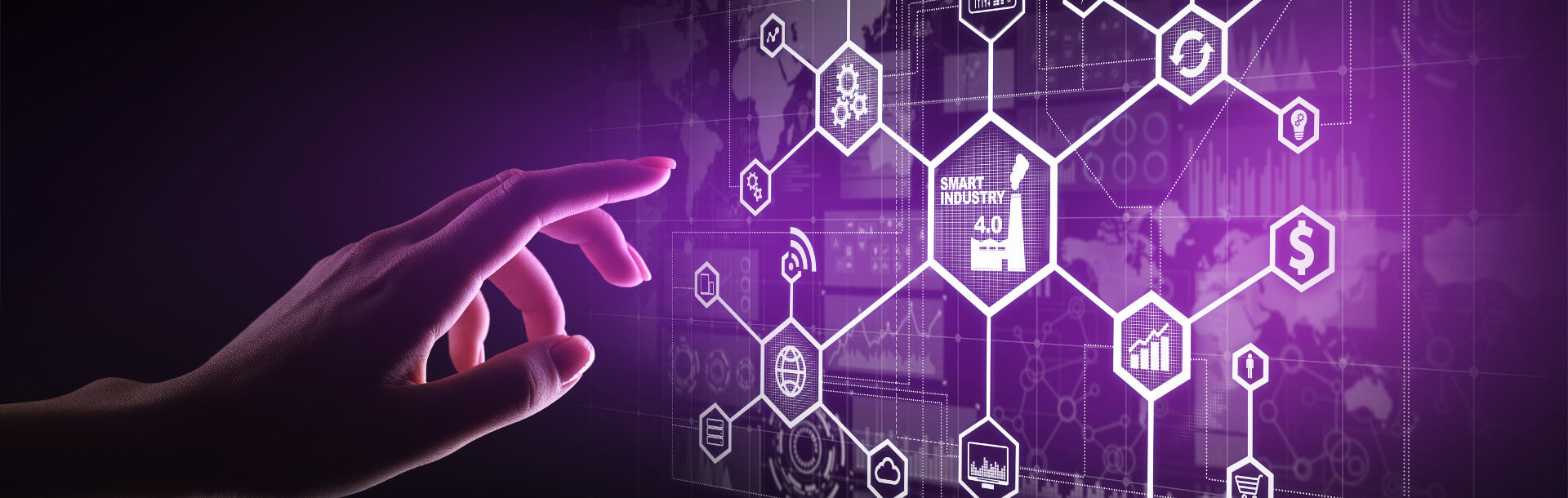
Advanced Food Image Recognition Technology by Deep Learning
Opportunity
The global image recognition market size was valued at nearly US$23 billion in 2018 and is projected to expand at a CAGR of 19% between 2018 and 2025. Within this rapidly-growing market is an emerging segment that combines image recognition technology and deep learning methods to automatically and accurately identify food from user-generated photos. Food recognition technology can be used to power mobile applications in the healthcare, F&B, and lifestyle industries. When deployed in healthcare and wellness apps, for example, food recognition technology can enable nutrition tracking and diet recommendations while, for F&B businesses, it can produce data and insights about the dishes that are popularly photographed and shared on social media.
Technology
This invention relates to an alignment and transfer network (ATNet) which is a deep learning-based approach for food recognition from user-generated photos. It uses privileged information (PI) to enhance conventional image-based food classification and is the first to combine knowledge graphs and item recommendation systems. Experimental comparison results suggest that it is able to achieve a comparatively higher accuracy than other algorithms.
The technology is based on a two-stage algorithm. After receiving food images and PI, which refers to any descriptive information for the corresponding images, the first stage includes a partial heterogeneous transfer method that aligns the features of the image and PI in the same space. It then applies statistical priors to model PI distribution under different food classes to enable a pipeline which uses the recovered PI for food classification. The technology then fuses food recognition results from two views—the conventional image classification and the classification using the recovered PI—to produce a more refined result. It can use any state-of-the-art convolutional neural network (CNN) model in the image channel and is able to consistently improve the CNN’s performance in terms of result accuracy.
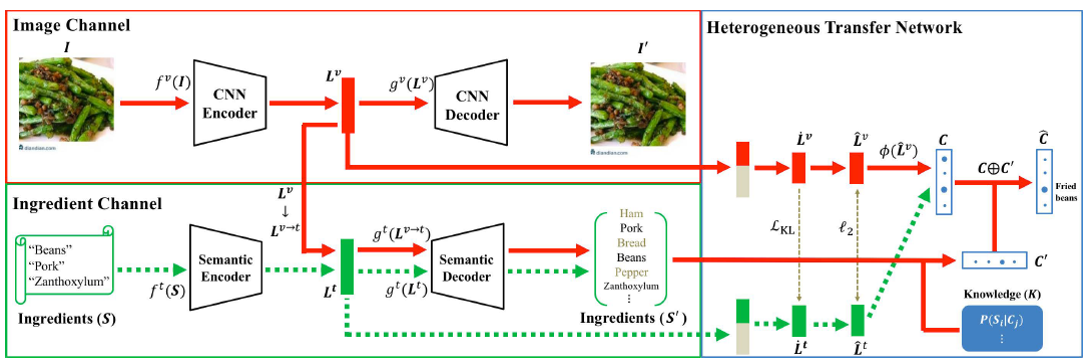
The framework of ATNet